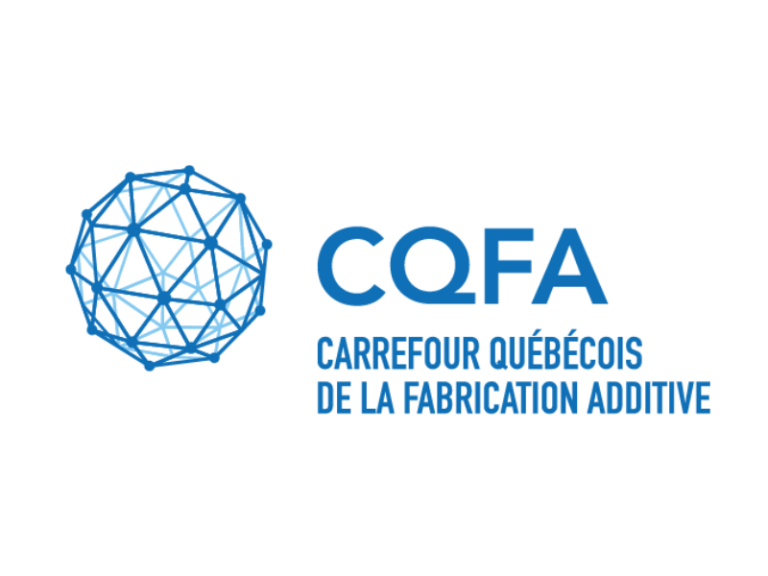
2023/02/13
Development of hybrid machine learning models for assessing the manufacturability of designs for additive manufacturing processes
Zhang, Y. (2022). Development of hybrid machine learning models for assessing the manufacturability of designs for additive manufacturing processes. Thesis.
Additive manufacturing (AM), which is also widely known as three-dimensional (3D) printing, has been a new trend in the manufacturing process in recent years. It can produce parts following a generated 3D model by adding layers of materials and fusing them. The main advantage of AM is the ability to enable customization and fabrication of complex geometries such as lattice structures, which are extremely difficult to manufacture in the subtractive manufacturing process. Although AM has been employed in many industrial applications, it is still difficult for beginning users to ensure the success of every print. It requires users to have a deep understanding of AM techniques to fully utilize this technology. The printing may fail owing to many factors such as the poor selection of the build orientation, materials, process settings, and insufficient geometric support for overhangs. It is difficult for non-AM experts to determine whether their designs are printable through a selected AM process, and it is even more difficult for them to make proper modifications without expert guidance before the fabrication. To fill these knowledge gaps, this study investigated the use of machine learning (ML) to assess the manufacturability of designs for AM processes. A web-based automated manufacturability analyzer and recommender for AM was developed as the implementation of the developed hybrid ML models. This tool can be used for the first-level evaluation of designs for novice AM users such as designers to ensure efficiency in terms of time and cost required for AM fabrications. The major contributions of this thesis are listed as follows: 1) Establishment of a unique database for the laser-based powder bed fusion (LPBF) process and fused deposition modeling (FDM) process. 2) Development of a novel approach on manufacturability analysis of LPBF using hybrid ML models. The models consider both process information and design perspectives. 3) Development of a hybrid sparse convolutional neural network (CNN) to predict manufacturability to increase the efficiency and effectiveness of the ML models. 4) Development of a recommendation system to provide potential modifications to assist users on AM printing. 5) A web-based application of analyzer and recommender was implemented to provide a comprehensive and easy-to-access manufacturability analysis to novice AM users. 6) Demonstration of how data-driven approaches can help on design and manufacturing processes and the framework can be extended to any process where parts can be classified based on visual inspection and basic labeling.